- 快召唤伙伴们来围观吧
- 微博 QQ QQ空间 贴吧
- 文档嵌入链接
- <iframe src="https://www.slidestalk.com/CarbonData/CarbonDatasparksummitchina201783653?embed" frame border="0" width="640" height="360" scrolling="no" allowfullscreen="true">复制
- 微信扫一扫分享
CarbonData 大数据交互式分析实践(spark summit)
展开查看详情
1 .CarbonData: 大数据交互式分析实践
2 . Agenda • 为什么需要CarbonData • CarbonData介绍 • 性能测试 • 应用案例 • 未来计划 2
3 . 企业中包含多种数据应用,从商业智能、批 处理到机器学习 Report & Dashboard OLAP & Ad-hoc Batch processing Machine learning Realtime Analytics Big Table Small table data Ex. CDR, transaction, Small table Web log,… Unstructured data 3
4 . 数据应用举例 过去1天使用Whatapp应用的终端按流量排名情况? 过去1天上海市每个小区的网络拥塞统计? Tracing and Record Query for Operation Engineer 4
5 . 来自数据的挑战 • Data Size 百亿级数据量 • Single Table >10 B • Fast growing • Multi-dimensional 多维度 • Every record > 100 dimension • Add new dimension occasionally • Rich of Detail 细粒度 • Billion level high cardinality • 1B terminal * 200K cell * 1440 minutes = 28800 (万亿) 5
6 . 来自应用的挑战 • Enterprise Integration 企业应用集成 • SQL 2003 Standard Syntax Multi-dimensional OLAP Query • BI integration, JDBC/ODBC 灵活查询 • Flexible Query 无固定模式 • Any combination of dimensions • OLAP Vs Detail Record Full Scan Query Small Scan Query • Full scan Vs Small scan • Precise search & Fuzzy search 6
7 . How to choose storage? 如何构建数据平台? 7
8 . 选择1: NoSQL Database Key-Value store: low latency, <5ms 只能通过Key访问,一键一值 适合实时应用对接,不适合分析型应用 8
9 . 选择2:Parallel database • Parallel scan + Fast compute 细粒度控制并行计算,适合中小规模 数据分析(数据集市) • Questionable scalability and fault-tolerance • Cluster size < 100 data node 扩展能力有上限 • Not suitable for big batch job 查询内容错能力弱 不适合海量数据分析(企业级数仓) 9
10 . 选择3: Search engine •All column indexed 适合多条件过滤,文本分析 •Fast searching •Simple aggregation •Designed for search but not OLAP 无法完成复杂计算 •Not for TopN, join, multi-level aggregation •3~4X data expansion in size 数据膨胀 •No SQL support 专用语法,难以迁移 10
11 . 选择4: SQL on Hadoop •Modern distributed architecture, scale well in computation. •Pipeline based: Impala, Drill, Flink, … 并行扫描+并行计算 适合海量数据计算 •BSP based: Hive, SparkSQL •BUT, still using file format designed for batch job 仍然使用为批处理设 计的存储,场景受限 •Focus on scan only •No index support, not suitable for point or small scan queries 11
12 . 适用场景 只针对某个场景设计,解决一部分问题 Option Store Good Bad KV Store HBase, Multi-dimensional OLAP Query Cassandra, … Parallel Greenplum, database Vertica, … Search Solr, engine ElasticSearch ,… SQL on Impala, Hadoop - HAWQ, Pipeline Drill, … Full Scan Query Small Scan Query SQL on Hive, Hadoop - SparkSQL BSP 12
13 . 架构师如何选择? Choice 1: Compromising Choice 2: Replicating of data 做出妥协,只满足部分应用 复制多份数据,满足所有应用 App1 App2 App3 App1 App2 App3 Loading Replication Data Data 13
14 .CarbonData目标: 一份数据满足多种业务需求,与大数据生态无缝集成 Multi-dimensional OLAP Query CarbonData: Unified Storage Full Scan Query Small Scan Query 一份数据满足多种分析场景 详单过滤,海量数仓,数据集市,… 14
15 . Apache CarbonData社区介绍 15
16 . Apache CarbonData • 2016年6月,进入Apache孵化器 Compute • 2016年9月,第一个生产系统部署 • 2017年4月,Apache毕业,成为Apache顶级项目 http://carbondata.apache.org • 共发布: Storage • 5个稳定版本 • 最新版本: 1.1.0 • 目前可访问CarbonData的计算引擎: • Spark, Presto, Hive, Flink 16
17 . Growing Community 每个月约100个JIRA 每个月约100个PR 每两个月新增约15个贡献者 •Diversity organization: •In production: 17
18 . 感谢社区用户的贡献 • Partition – 上汽集团 • Bitmap Encoding – 上汽集团 • Presto Integration – 携程 • Hive Integration – 滴滴、Knoldus 18
19 . CarbonData + Spark: 打造大数据交互式分析引擎 19
20 . CarbonData大数据生态 • 支持Spark、Hive、Presto、Flink • 内置Hadoop和Spark深度优化 • Hadoop: > 2.2 Data Query • Spark 1.5, 1.6, 2.1 Management Optimization • 接口 • SQL Read/Write/Update • DataFrame API • 支持操作: Carbon File Carbon File Carbon File • 查询:支持SparkSQL优化器 • 数据管理:批量入库、更新、删除、合并 (Compaction)、增删列 20
21 .使用方式:入库 • SQL CREATE TABLE tablename (name String, PhoneNumber String) STORED BY “carbondata” TBLPROPERTIES (...) LOAD DATA [LOCAL] INPATH 'folder path' [OVERWRITE] INTO TABLE tablename OPTIONS(...) INSERT INTO TABLE tablennme select_statement1 FROM table1; • Dataframe df.write .format(“carbondata") .options("tableName“, “t1")) .mode(SaveMode.Overwrite) .save() 21
22 .使用方式:查询 • SQL SELECT project_list FROM t1 WHERE cond_list GROUP BY columns ORDER BY columns • Dataframe df = sparkSession.read .format(“carbondata”) .option(“tableName”, “t1”) .load(“path_to_carbon_file”) df.select(…).show 22
23 .使用方式:更新和删除 Modify one column in table1 UPDATE table1 A phone, 70 60 SET A.REVENUE = A.REVENUE - 10 car,100 WHERE A.PRODUCT = ‘phone’ phone, 30 20 Modify two columns in table1 with values from table2 UPDATE table1 A SET (A.PRODUCT, A.REVENUE) = ( SELECT PRODUCT, REVENUE FROM table2 B table1 table2 WHERE B.CITY = A.CITY AND B.BROKER = A.BROKER ) WHERE A.DATE BETWEEN ‘2017-01-01’ AND ‘2017-01-31’ Delete records in table1 DELETE FROM table1 A 123, abc WHERE A.CUSTOMERID = ‘123’ 456, jkd 23
24 . CarbonData介绍 CarbonData CarbonData 文件 表格 数据管理 查询 24
25 . CarbonData介绍 CarbonData CarbonData 文件 表格 数据管理 查询 25
26 . CarbonData文件格式 Carbon Data File •Blocklet: 文件内的数据块 File Header •Data are sorted along MDK (multi-dimensional keys) Version •Clustered data enabling efficient filtering and scan Schema •Column chunk: Blocklet内的列数据 Blocklet 1 Column 1 Chunk •一次IO单元 Page1 Page2 Page3 … •内部分为多个Page,解压单元 Column 2 Chunk … •元数据和索引信息 Column n Chunk •Header:Version,Schema … •Footer: Blocklet Index & statistics Blocklet N •内置索引 File Footer •Multi-dimensional Index Blocklet Index & Stats •Statistics: Column Min/Max, Cardinality 26
27 .索引建立过程介绍 Years Quarters Months Territor y Countr y Quantity Sales 2003 QTR1 Jan EMEA Germany 142 11,432 2003 QTR1 Jan APAC China 541 54,702 2003 QTR1 Jan EMEA Spain 443 44,622 •数据和索引合一存储,数据即索引 2003 QTR1 Feb EMEA Denmark 545 58,871 •多维索引 (Multi-Dimensional Key) 2003 2003 QTR1 QTR1 Feb Mar EMEA APAC Italy India 675 52 56,181 9,749 •全局字典编码 2003 2003 QTR1 QTR1 Mar Mar EMEA Japan UK Japan 570 561 51,018 55,245 2003 QTR2 Apr APAC Australia 525 50,398 2003 QTR2 Apr EMEA Germany 144 11,532 Blocklet (Columnar view) Sorted MDK Index Encoding C1 C2 C3 C4 C5 C6 C7 1 1 1 1 1 142 11432 [1,1,1,1,1] : [142,11432] [1,1,1,1,1] : [142,11432] 1 1 1 1 3 443 44622 [1,1,1,1,3] : [443,44622] [1,1,1,3,2] : [541,54702] 1 1 1 3 2 541 54702 [1,1,1,3,2] : [541,54702] [1,1,1,1,3] : [443,44622] 1 1 2 1 4 545 58871 1 1 2 1 5 675 56181 [1,1,2,1,4] : [545,58871] [1,1,2,1,4] : [545,58871] 1 1 3 1 7 570 51018 [1,1,2,1,5] : [675,56181] [1,1,2,1,5] : [675,56181] 1 1 3 2 8 561 55245 [1,1,3,1,7] : [570,51018] Sort [1,1,3,3,6] : [52,9749] 1 1 3 3 6 52 9749 [1,1,3,2,8] : [561,55245] (MDK Index ) 1 2 4 1 1 144 11532 [1,1,3,1,7] : [570,51018] 1 2 4 3 9 525 50398 [1,1,3,3,6] : [52,9749] [1,1,3,2,8] : [561,55245] [1,2,4,1,1] : [144,11532] [1,2,4,3,9] : [525,50398] [1,2,4,3,9] : [525,50398] [1,2,4,1,1] : [144,11532] 27
28 .索引建立过程介绍(二) Blocklet File Footer Blocklet 1 Blocklet Index • Build in-memory file level MDK index tree for 1 1 1 1 1 1 12000 filtering 1 1 1 2 1 2 5000 Blocklet1 1 1 2 1 1 1 12000 • Major optimization for efficient scan 1 1 2 2 1 2 5000 Start Key1 1 1 3 1 1 1 12000 End Key1 1 1 3 2 1 2 5000 Start Key1 Blocklet 2 C1(Min, Max) 1 2 1 3 2 3 11000 End Key4 1 2 2 3 2 3 11000 …. 1 2 3 3 2 3 11000 1 3 1 4 3 4 2000 C7(Min, Max) Start Key1 1 3 1 5 3 4 1000 Start Key3 1 3 2 4 3 4 2000 End Key2 End Key4 Blocklet 3 1 3 2 5 3 4 1000 1 3 3 4 3 4 2000 Start Key1 Start Key2 Start Key3 Start Key4 1 3 3 5 3 4 1000 Blocklet4 1 4 1 4 1 1 20000 End Key1 End Key2 End Key3 End Key4 1 4 2 4 1 1 20000 Start Key4 1 4 3 4 1 1 20000 C1(Min,Max) C1(Min,Max) C1(Min,Max) C1(Min,Max) Blocklet 4 End Key4 2 1 1 1 1 1 12000 … … … … 2 1 1 2 1 2 5000 C1(Min, Max) C7(Min,Max) C7(Min,Max) C7(Min,Max) C7(Min,Max) 2 1 2 1 1 1 12000 …. 2 1 2 2 1 2 5000 2 1 3 1 1 1 12000 C7(Min, Max) 2 1 3 2 1 2 5000 28
29 . 利用两级索引架构减少Spark Task数和磁盘IO Spark Driver • 第一级:文件级索引 用于过滤文件(HDFS Block), Catalyst 避免扫描不必要的文件,减少多 Block Index 达95%的Spark Task Executor Executor • 第二级:Blocklet索引 Blocklet Index Blocklet Index 用于过滤文件内部的Blocklet, 避免扫描不必要的Blocklet,减 少磁盘IO Carbon File Carbon File Carbon File Data Data Data Footer Footer Footer 29
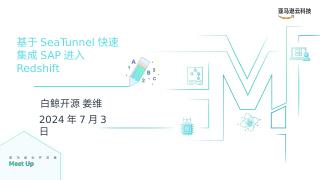
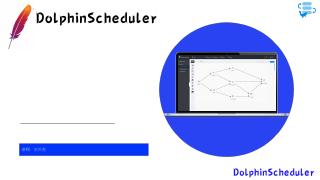
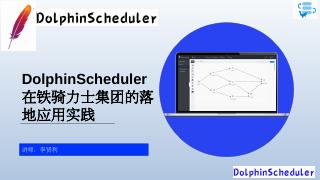
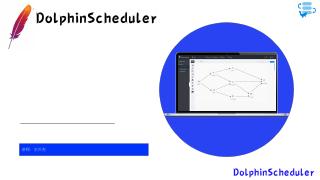
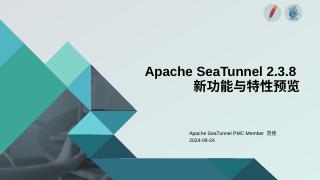
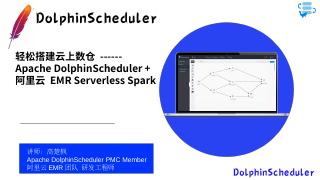
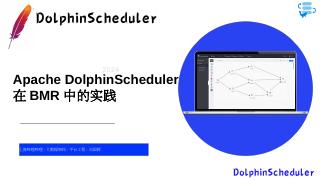
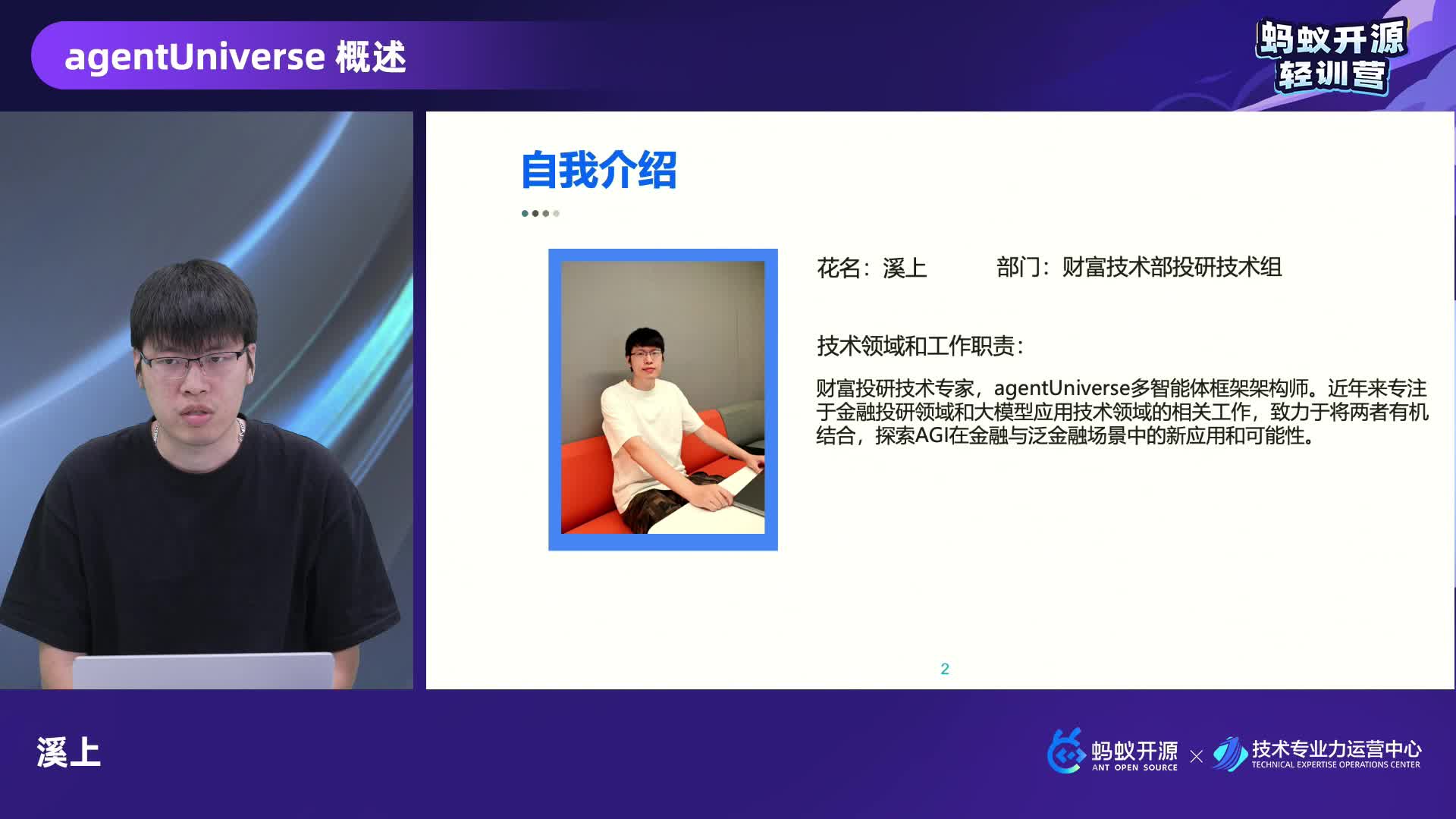