- 快召唤伙伴们来围观吧
- 微博 QQ QQ空间 贴吧
- 视频嵌入链接 文档嵌入链接
- <iframe src="https://www.slidestalk.com/Spark/MachineLearningatScalewithMLflowandApacheSpark74772?embed&video" frame border="0" width="640" height="360" scrolling="no" allowfullscreen="true">复制
- 微信扫一扫分享


Machine Learning at Scale with MLflow and Apache Spark
Societe Generale is one of the major banks in France and has many data science teams across the globe. After years of explorations and prototyping, it is time for the company to really deploy machine learning projects at scale to the production environment.
To achieve that goal, we have been working hard to define a standard process of collaboration between data engineers and data scientists. And we also designed and deployed an infrastructure for productionizing machine learning.
During this presentation, you will be looking at the following points of our adventure:
- Difficulties that we had for putting ML applications into production, such as lack of model registry; hard to deploy ML libraries to our Hadoop cluster; collaboration between data scientists and data engineers etc. ?
- How did we deploy MLflow as a key technical component to our production hadoop environment given different security constraints.
- How did we build a CI/CD pipeline to deploy ML applications automatically. MLflow plays an important role in this piepline.
- A first and concrete production project developed on top of this infrastructure with MLflow, Spark streaming, Sklearn and CI/CD.
The key takeaways of this presentation would be:
- To productionize machine learning in a big structure like Société Générale, a process of collaboration should be clearly defined.
- A ML model registry is key to ML productionization. MLflow is the best solution we found.
- A CI/CD pipeline is essential to the success of a machine learning application.
展开查看详情
1 .WIFI SSID:Spark+AISummit | Password: UnifiedDataAnalytics
2 .Machine learning at scale with MLflow and Spark Chongguang LIU, Société Générale #UnifiedDataAnalytics #SparkAISummit
3 .About me • Studied computer science and engineering • Data engineer at SocGen • Using Spark and MLflow at work • Skiing and diving during vacations #UnifiedDataAnalytics #SparkAISummit 3
4 .Data is strategic at SocGen • SocGen is French multinational bank. • We have 80+ data pipelines in production in our data lake. • More than 200 data scientists working across the globe. • Data allows us to create new products, improve customer experience and be more efficient. • Relevant use cases such as anti-money laundering, fraud detection, automatic document analysis etc. 4
5 .But also a lot of pain points ... ine s s ! for bus d v a lue Difficult to use ML models Suboptimal predictions No automated data flow Manually deploy models Lim ite Models rarely updated Manually copy training data Code rewrite in another programming language Business Data scientist Data engineer 5
6 .Finally we realised that ... ML Code Hidden technical debt in machine learning systems, 2015, Google 6
7 .Challenge 1: data locality • A central Hadoop cluster • Client data, transaction data, accounting data etc. • Automated data pipelines • Banking industry is highly regulated, sensitive data is kept in the data lake for security reasons. training and prediction inside the data lake 7
8 .Challenge 2: application reliability prototyping phase 8
9 .Challenge 2: application reliability production phase Yarn Data node Data node Data node 9
10 .Challenge 3: variant python packages python code python code python code + + conda env conda env 10
11 .Challenge 4: model management 11
12 .Challenge 4: model management MLflow tracking server HDFS ML meta data ML models Data node Data node Data node 12
13 .Challenge 5: tracking server reliability Tracking server Data node HA proxy PostgreSQL Tracking server 13
14 .Challenge 6: model serving Kafka Knox Spark streaming API server MLflow HDFS 14
15 .A concrete example news news model MLflow ML model server score Kafka Spark streaming score new model DB feedback score score feedback HDFS Spark + Sklearn feedback Web app periodic model retraining 15
16 .Moving forward • Model drift monitoring • A/B testing • pandas_udf • koalas 16
17 .Thank you! 17
18 .DON’T FORGET TO RATE AND REVIEW THE SESSIONS SEARCH SPARK + AI SUMMIT
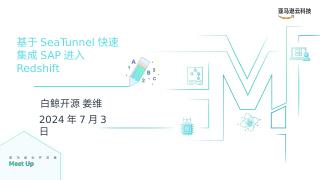
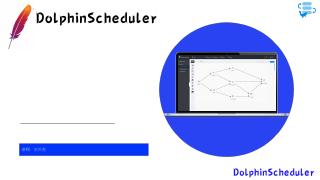
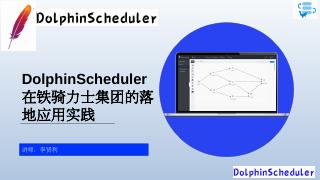
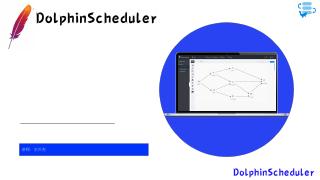
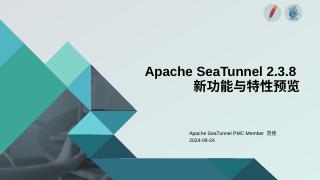
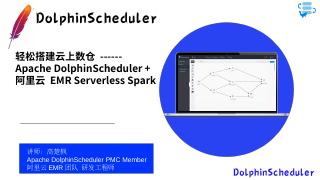
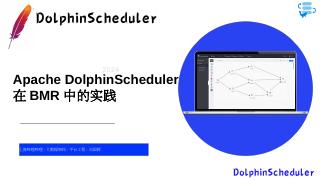
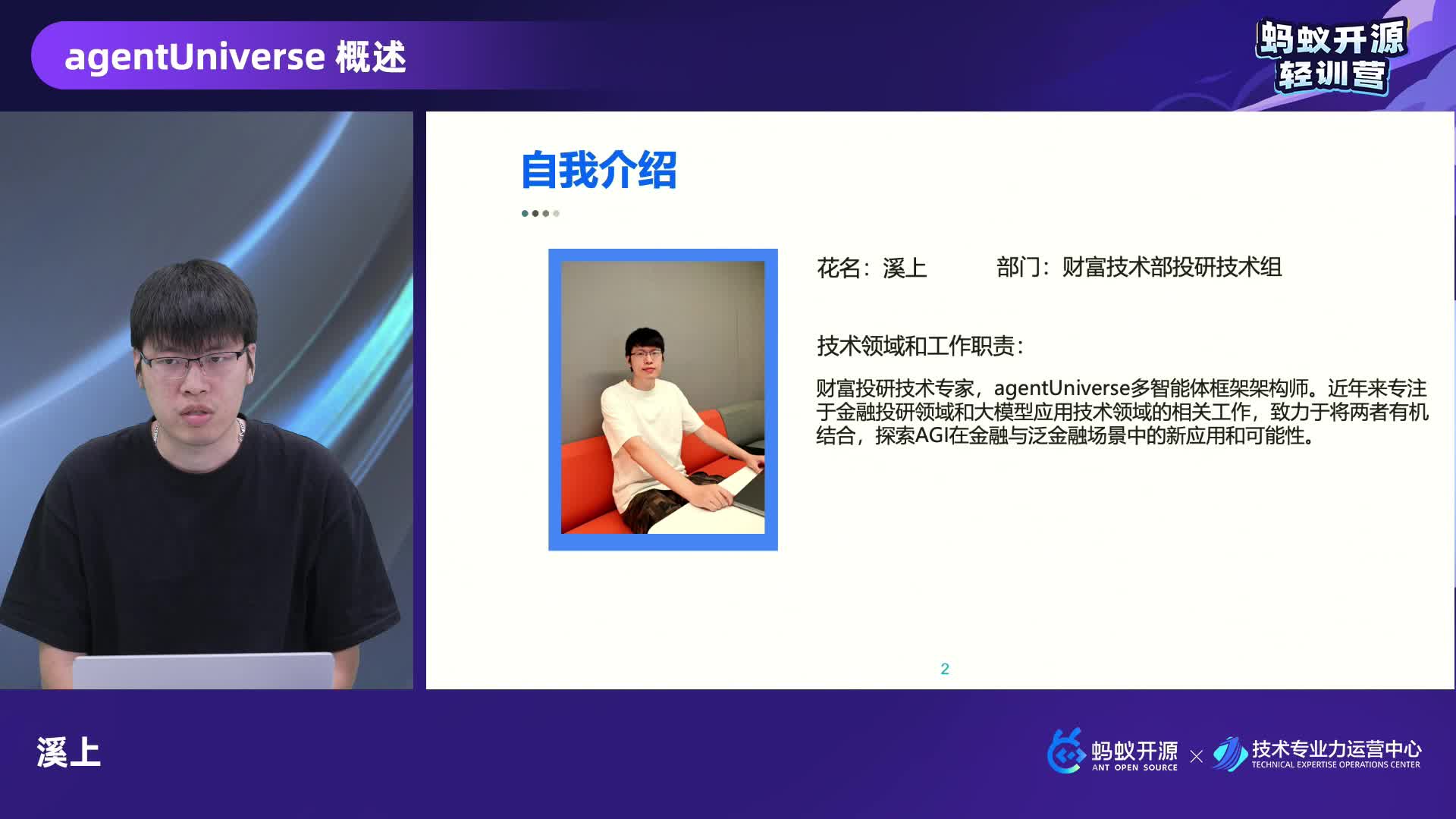